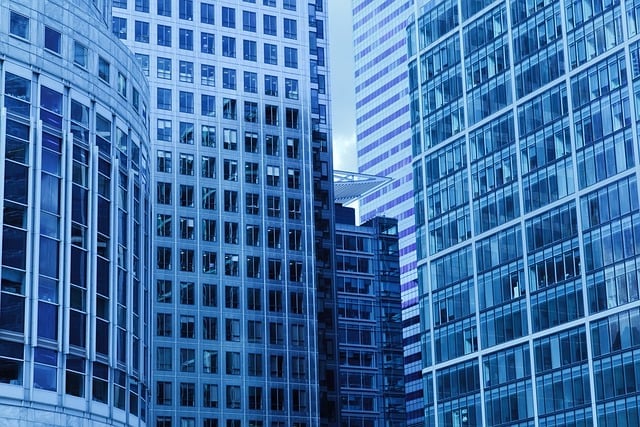
ATO Speech at UNSW 16th ATAX Conference
Jeremy Hirschhorn, Second Commissioner, Client Engagement Group
Speech delivered at the UNSW 16th ATAX International Conference
on Tax Administration
Sydney, 8 April 2025
(Check against delivery)
Thank you for having me today.
In reflecting on this topic and preparing for today, I have realised the real topic I would like to discuss is trust:
So today, I will only touch on some of the actual uses of artificial intelligence (AI) and automation by the ATO. The focus will be on how a tax administrator should approach its duty to be trustworthy in the area of data, automation and AI.
Good use of AI starts with a strong culture of ethical stewardship of all data use and sharing. This includes an ethical approach to transparency about how you are storing the data and the safeguards in place to protect it, and crucially, the ethical administration of systems.
The ATO has a range of formal governance arrangements in place for use of data in the organisation, as well as a number of APS-wide ones we align our practices to. We’ve developed further guidelines including Chief executive instructions for our staff, and the ATO data ethics principles which are published on our website as our public commitment to Australian taxpayers. They lay out the protocols that govern how we collect and store data, what it’s used for, and who the data is shared with. The 6 data ethics principles are worth briefly highlighting for you here:
Underpinning good decision making (whether by carbon or silicon!) is high quality data. The ATO has some of Australia’s largest data holdings, and we invest heavily in the quality of that data and work hard to make sure it’s usable.
Without good data, you won’t get too far, in fact, you’ll probably go far in the wrong direction.
Everyday Australians trust us to acquire and hold their private financial information. Importantly, this sharing is not freely chosen by individuals, but is compulsory.
Further, in the context of information obtained under compulsory powers, taxpayers must provide us information even if that information would be self-incriminating. This particular exception to the general rule in a liberal democracy is justified on the basis that some financial information is uniquely in the possession of the taxpayer, and the job of a tax administrator could be easily frustrated without this exception.
These factors emphasise the sensitivity and care with which we must treat taxpayer data. On-sharing of this data, even with other parts of Government, must be strictly in accordance with law. But perhaps more importantly, and a lesson from Robodebt, is that the tax administrator must continue to act as a steward of that data even after it has been legally shared.
It is very important to make sure your use of data takes into account its quality and reliability.
We now tend to think of data as on a curve:
Importantly, before making any decision based on data, it is critical to understand the potential impact on the taxpayer of the tax administrator making a mistake, and to ensure that you have the procedural and cultural safeguards to protect against ‘high impact actions’ made in error.
This focus on potential errors is very hard. It forces you to understand the other person’s world (and how your actions may affect it). Thinking about errors requires a discipline as classic measures such as complaint levels or error rates do not get to the heart of whether your errors are impactful or not. Being a data-driven organisation arguably exacerbates (rather than improves) this challenge – it is all too easy to fall in the trap of ‘data hubris’.
Ideally these potential errors are identified while they are still ‘potential’. However, a tax administrator must remain hyper-vigilant. Noting that most people are fundamentally honest, a high ‘hit rate’ should be viewed with great caution. It is more likely to be a sign of ‘data hubris’ than widespread non-compliance, and should be treated as such until proven otherwise. The UK Post Office scandal is a prime example of an institution having excessive trust in the computer systems and insufficient trust in ordinary people.
AI may be a helper. It can move things around, it can link, synthesise and analyse information, and it can do some things much faster and more consistently than we as humans can. But AI cannot determine what constitutes fairness and reasonableness, having considered unique taxpayer circumstances with compassion and empathy. (And, in my experience, perhaps most dangerously, AI doesn’t know when to say it doesn’t know). AI should be thought of as a bionic arm. It’s an extension of our thinking and our actions; a tool – but not a replacement.
What this means is that any decision which adversely affects the rights of taxpayers should be made by a human.
But further, I would posit that, even in some future where AI passes some form of advanced Turing’s test for compassion and empathy, part of the social compact with citizens is that they want a human to make decisions with important impacts on their life.
This does not mean that the use of automation and AI is limited to ‘service’, but ‘service’ enabled by automation and AI, such as pre-fill, is of extraordinary value to citizens in making their lives easier. Automation and AI can be very useful for risk analysis and case selection: for analysing documents for key information to support auditors getting to the heart of a matter quickly, and for nudging taxpayers in real time when they may be taking unwise actions.
I would further posit that another element of the trust equation (at least for a tax administrator, if not every Government and large organisation) is that actions or decisions should be explicable by a human to the affected person in a way that the affected person can understand (even if automated or performed by AI). If you do not know why your organisation is doing things (‘the computer said so’), you are breaching your responsibility to be accountable to both the individual taxpayer, but also the broader system.
Building on the ‘data hubris’ point, automation and AI will reflect and possibly amplify previous hidden biases (whether you are a public or private sector organisation). An example of this was the Dutch child care scandal, where the risk rules underpinning an anti-fraud compliance program were found to be biased against non-citizens.
Again, bias is a very tricky thing for individuals and institutions to self-identify, so it is important to be vigilant about possible implicit biases leading to systemic issues.
Of course, the biases can be hiding in the original training set, but importantly can also arise from how you ‘train’ the AI on an on-going basis. I remember reading an article, probably 25 years ago, entitled “Is your spreadsheet a tax evader?”. The article was based on 2 premises:
Where there is an unpleasant surprise, people will dig into it and find and fix the underlying bug. But where there is a pleasant surprise, people will be much less diligent in working out why (which means ‘pleasant’ bugs remain, but ‘unpleasant’ bugs are weeded out, so over time the tax spreadsheet will systemically understate tax payable).
Similar risks apply to training an AI model. If your users/trainers only query ‘unpleasant’ results (from their perspective), the model will gradually skew, even if it started off unbiased. A tax administrator must be careful that their AI does not get progressively more defensive of the revenue, but similarly that a private sector tax AI model does not evolve into an aggressive tax planner!
There is a strong temptation for a tax administrator to take on more and more data, a temptation strengthened in the era of AI, which can feed off sprawling data sets.
It has often been said that ‘data is gold’ or ‘data is the new oil’. But I would say that ‘data is uranium’ (I wish I had coined this, but I have taken it from others). Before you get it you better know how you’re going to use and store it and there needs to be very good reasons to take the risk!
I would also say that, as a tax administrator in a liberal democracy, and as part of the trust equation, the usefulness of the data must be measured against the intrusiveness of the request. Taking on data ‘just in case’, or because it might be handy for AI analysis will not pass the test.
In fact, I would argue the opposite – that AI and digitalisation can enable tax administration with less intrusive data collection. In other words, as taxpayers are increasingly digitalised, a tax administrator should explore moving their administration (risk engines, etc.) to the taxpayer’s natural systems (and data), rather than needing to acquire and hold all that data. The further advantage of this philosophy is that it helps taxpayers to minimise their chance of making a mistake and coming to our attention.
In my earlier points I urged caution about automation and AI. But this is in the context that it is now part of the core function of a tax administrator, from both service and compliance perspectives, as well as the efficient use of the resources provided to a tax administrator to acquit its duties.
Do not focus so much on the risk of doing things, that you ignore the risk of not doing things!
I have emphasised above that, before embracing automation and AI, it is necessary to get your data settings in order. For a period, you can rely on your governance around data and IT systems. At some point (probably now or soon), automation and AI become so critical that you can no longer rely on those governance frameworks, but need specific governance.
And finally, just in case, be nice to Siri, she may have a long memory …
https://www.ato.gov.au/media-centre/speech-to-unsw-16th-atax-international-conference