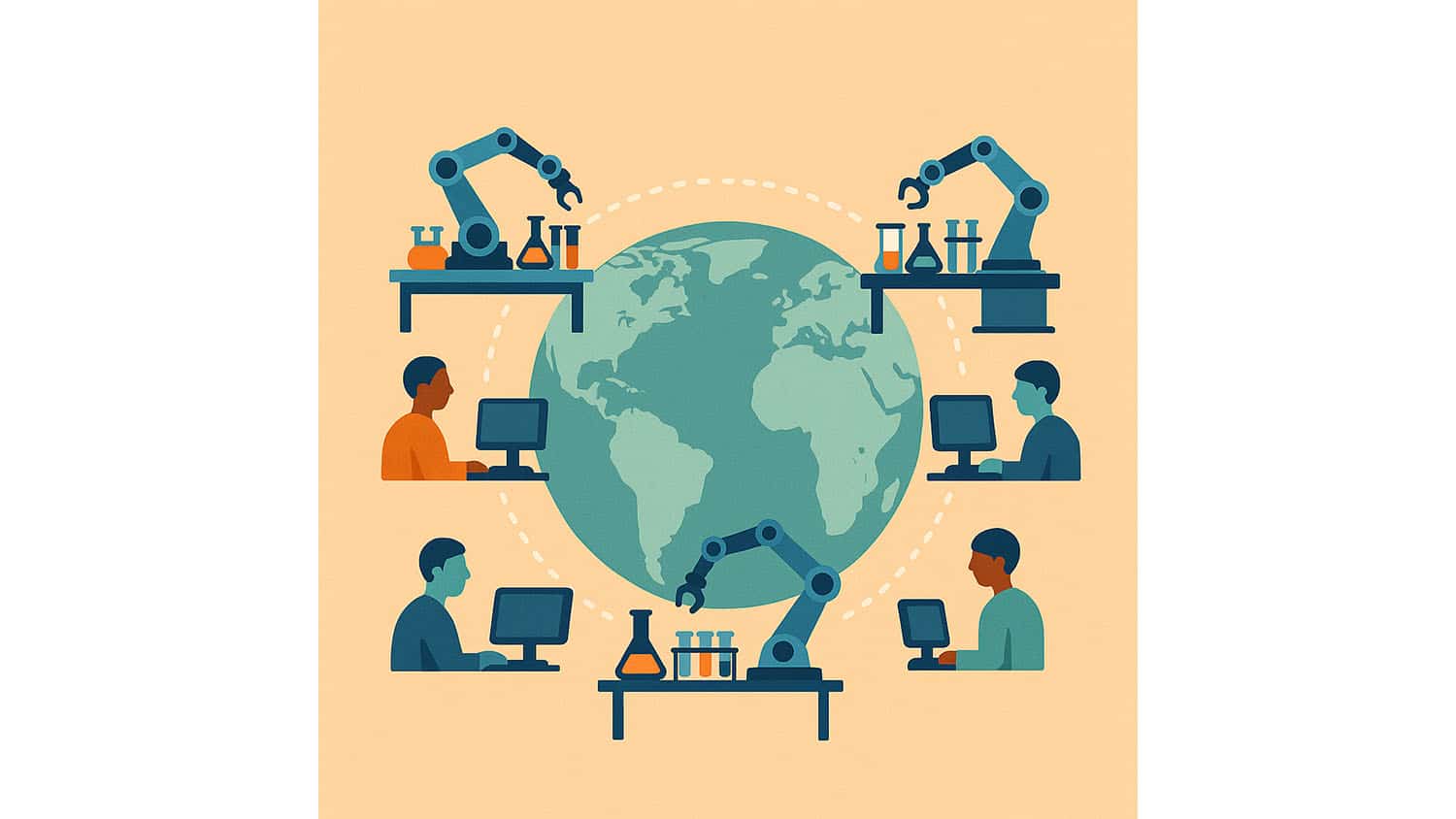
Self-Driving Labs: New Era in Scientific Research?
An interdisciplinary team of scientists and engineers is arguing the research community should make a concerted effort to capitalize on advances in robotics and artificial intelligence (AI) to expedite work that addresses sustainable energy, emerging diseases and other global challenges.
“Self-driving labs (SDLs) will serve as collaborators for human researchers, significantly reducing the time and cost required to reach scientific solutions,” says Milad Abolhasani, an expert in the field and ALCOA Professor of Chemical and Biomolecular Engineering at North Carolina State University. “They will not replace the unique expertise or creativity of human researchers. Instead, these advanced technologies will streamline tedious and repetitive experimental tasks, automate data analysis, and assist in informed decision-making. This approach enables researchers to rapidly explore new ideas, push the boundaries of innovation and significantly accelerate scientific discovery.”
Abolhasani and a dozen colleagues published a piece in Nature Communications April 24 laying out a blueprint for how SDLs can expedite valuable research – and how a new approach to these tools could make the technology more accessible and accelerate research in critical areas.
We talked to Abolhasani about the argument he and his collaborators are making in favor of SDLs, and what the path forward may look like.
The Abstract: What is a self-driving lab?
Milad Abolhasani: An SDL is essentially a robotic co-pilot for scientific research. It automates the entire scientific research process – from designing experiments and executing them to analyzing results. Equipped with AI, robotics and automation, SDLs dynamically learn and adapt based on experimental outcomes, continuously improving their methods through a closed-loop process. Think of it as an AI-powered robotic collaborator that helps scientists rapidly explore new ideas, streamline research workflows and accelerate groundbreaking discoveries.
TA: What is the big idea that you and your collaborators are proposing in this paper?
Abolhasani: In our recent article, published in Nature Communications, we (12 other SDL researchers from 11 institutions across the United States and Europe and I) discuss the massive potential of SDLs to enable streamlined team science and accelerate scientific discoveries on a global scale.
We highlight how SDLs can dramatically enhance collaboration and innovation across different scientific disciplines. Additionally, we address existing roadblocks to making this vision a reality and propose strategic solutions, including a coordinated effort between centralized SDL facilities and distributed lab networks. To achieve this ambitious goal, we emphasize the necessity of prioritizing federal and industrial investments, alongside targeted academic research, to build reliable, accessible and robust SDL infrastructure.
TA: Can you give me examples of real-world problems or scientific advances that SDLs have already addressed? Or of challenges that self-driving labs are exceptionally well-suited to tackle?
Abolhasani: SDLs have already demonstrated significant reductions in the time and cost required to achieve solutions in critical areas such as energy, the environment and health care. For example, SDLs have accelerated research breakthroughs in battery technologies, solar cell development, pharmaceuticals, specialty materials and wearable electronics, achieving discoveries 10 to 100 times faster than traditional methods.
With further advancements, SDLs have the potential to accelerate research by as much as 1,000 times, making them exceptionally well-suited to tackling complex, multidimensional problems that involve extensive experimentation.
TA: Okay, let me step back for a second. Broadly speaking, what are the benefits SDLs could provide for the research community? Why would researchers want to use these technologies?
Abolhasani: Researchers are increasingly turning to SDLs as robotic collaborators capable of navigating high-dimensional experimental spaces with exceptional efficiency. SDLs enable rapid hypothesis testing, iterative refinement of experimental strategies and intelligent exploration of complex parameter landscapes. These systems accelerate the research process, reduce experimental costs and minimize human error by automating labor-intensive and repetitive tasks. By offloading these burdens, SDLs allow researchers to focus on higher-order scientific questions and creativity, facilitating modern, cloud-connected team science at an unprecedented pace and scale.
TA: You and your collaborators talk about a coordinated effort that would include both centralized research facilities and a distributed network of labs that work separately. What’s the advantage of this approach?
Abolhasani: Combining centralized SDL facilities with distributed labs ensures both high-performance capabilities and broad accessibility. Centralized SDLs can handle complex, resource-intensive research, while distributed labs offer flexibility and ease of access, enabling scientists everywhere to actively participate in cutting-edge research.
TA: Why is this important? And why are you and your collaborators putting this idea forward now?
Abolhasani: A coordinated effort for both centralized and distributed SDLs is important because current global challenges – such as energy shortages, rapidly emerging diseases and urgent environmental crises – require solutions within months rather than decades. Fast-tracked scientific solutions are critical, and SDLs are uniquely positioned to meet this urgent need. We’re proposing this concerted effort now because recent breakthroughs in AI, robotics and lab automation have made it technically achievable, while the global challenges we are facing make it urgently necessary to rapidly deploy these advanced capabilities.
TA: What are the most significant challenges to moving forward with this vision of science acceleration and accessibility with SDLs?
Abolhasani: Key challenges include ensuring reliable hardware and technology integration, which means creating robust, interoperable systems that can consistently execute complex experimental workflows with minimal downtime and high precision. Seamless integration of software and hardware components is essential to support adaptive automation and reproducibility across diverse research applications.
Another foundational requirement is the generation and management of large volumes of high-quality data. AI models that drive SDLs rely heavily on these data for training, prediction and continuous improvement. Without rigorous data quality standards and consistent metadata practices, the reliability and generalizability of SDL outputs are compromised.
Additional challenges include establishing standards for knowledge transfer and augmentation. This refers to creating shared protocols, formats, and ontologies that allow insights, experimental strategies, and learned models from one SDL to be easily transferred, reused, or built upon by others. Such standards would ensure seamless collaboration and integration across different platforms and disciplines, making it possible for SDLs to augment each other’s capabilities and accelerate team science.
Additionally, developing a skilled workforce capable of designing, maintaining and operating these sophisticated platforms remains essential. The current SDL landscape is fragmented, with many efforts focused on application-specific hardware and software. To truly enable scalable, collaborative team science, a concerted shift is needed toward developing application-agnostic SDL systems that can serve as flexible, interoperable platforms across a range of disciplines.
Finally, legal and safety standards must be carefully considered to ensure responsible deployment and long-term sustainability of SDL technologies.
TA: Can you explain more about what you mean regarding legal and safety standards?
Abolhasani: Legal and safety standards refer to the policies, certifications and best practices needed to ensure that SDLs operate within regulatory frameworks while maintaining laboratory safety and compliance. This includes safe handling of hazardous materials, traceable data practices, responsible use of AI and clear guidelines for intellectual property rights. Establishing these standards is essential for building trust in SDL technologies and enabling their widespread and responsible adoption.
TA: So, what are the next steps? Where do you start?
Abolhasani: The next steps involve forming strategic, large-scale partnerships among academia, industry, and government agencies to align efforts, establish universal standards, and secure funding for reliable SDL infrastructure. Industry partnerships should include both equipment manufacturers and end-users of SDL technologies. Additionally, early pilot programs and dedicated testbeds demonstrating the effectiveness of centralized and distributed SDL models will be critical in validating and refining both centralized and distributed SDLs.
TA: If everything goes perfectly, how do you see this playing out over the next 10 years?
Abolhasani: In an ideal scenario, within the next decade SDLs will become a mainstream science collaborator – a robotic co-pilot – for researchers around the world, fundamentally changing the way we approach scientific research. They will drive rapid innovation, enhance global scientific collaboration and dramatically accelerate breakthroughs, making it possible to solve complex and urgent global challenges in areas such as environmental sustainability, energy efficiency and personalized health care much faster than ever before.
https://news.ncsu.edu/2025/04/self-driving-labs-new-era-of-research/